Driving Excellence
in the Manufacturing
Industry with AI and Analytics
Nurturing Smart Manufacturing with Affine
Services & Solutions across the Manufacturing Value Chain
Production Planning & Forecasting
Optimize Production Schedules and Forecast Demand Accurately
Labor Optimization
Optimize Labor Operations to Meet Production Demands
Predictive Maintenance
Anticipate Equipment Failures and Optimize Maintenance Schedules
Quality Assurance
Maintain Consistent Product Quality Using ML Models
Document Summarization
Summarize Daily Action Slips and Documents Accurately using our ML models
CAD/CAM Benchmarking
Streamline Production Processes and Enhance Quality Control of 3D Manufacturing Designs
Connected Factory Solutions
Increase Production Efficiency, Reduce Operational Costs, and Enhance OEE
Product Feature Recommendations
Enhance Overall Product Development and Provide Precise Product Recommendations
Inventory Management
Optimize Inventory Management and Improve Overall Supply Chain Efficiency
Supplier Risk Assessment Framework
Ensure Accurate Supplier Contracts to Avoid Potential Supplier Risks
Network & Route Scheduling
Minimize Transit Time and Costs Using Dynamic Route Optimization
Fees, Fines & Claims Auto Validation
Reduce Financial Loses due to Invalid Fines by Retailers Via Azure Open AI and Prompt Engineering Frameworks
Cost of Goods Sold
Ensure Maximum Yield and Minimum Wastage with Controlled Planning using Scalable Designs
Process and Asset Level Energy Monitoring
Monitor and Optimize Real-time Energy Consumption through Customizable Solutions
Energy Load Estimation & Consumption Summary Generation
Optimize Energy Usage and Reduce Costs Using Integrated and Scalable Systems
Real-time Agentic Recommendations
Improve Operational Efficiency with Actionable Recommendations for Real-time Processes
Defect Detection
Enhance Overall Productivity by Reducing Manual Intervention through Deep Learning Models
Service Contract Management
Enhance Employee Satisfaction through Fair Wages and Labor Dispute Management
Connected Field Service
Enhance Maintenance Support Using Scalable IoT Systems with Edge Computing Models
Quality Audit
Enhance Compliance Standards with Automated Processes and Scalable Solutions
Predictive Maintenance
Reduce Downtime and Minimize Maintenance Costs Using Scalable Cloud-Based Architecture
MRO Support & Recommendation
Enhance Decision-Making Capabilities through Detailed Insights and In-Depth Analysis
Services & Solutions across the Manufacturing Value Chain
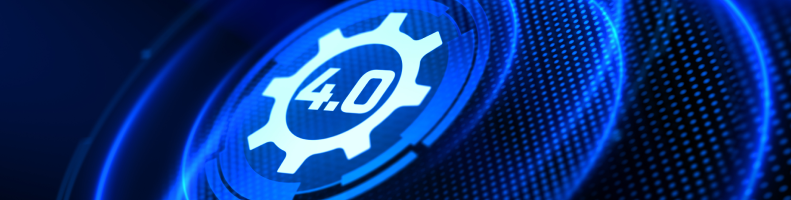
Customer Lifecycle Management
Optimize Marketing Spend with Precision
Personalized Recommendations
Boost Sales with Personalized Recommendations
Creative Operations
Enhance Marketing Efficiency with AI-Powered Automation
Microservices Design & Development
Elevate Retail Operations with Microservices
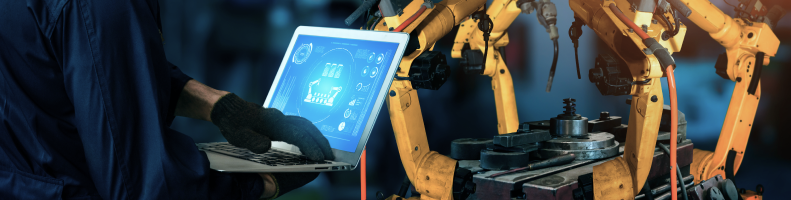
Employ production optimization strategies like automation systems, robotics management, etc., using our data intelligence and IoT models.
Detect equipment failures and include efficient quality control measures to reduce downtime using our AI-powered predictive maintenance models.
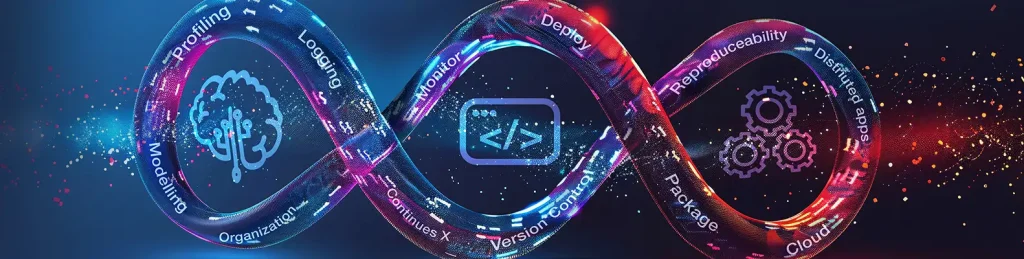
Identify recipe insight strategies, standardize raw material quantities, and streamline manufacturing processes using our ML and BI models.
Efficiently manage transportation, warehouse, and overall logistics using our advanced analytics and AI/ML models.
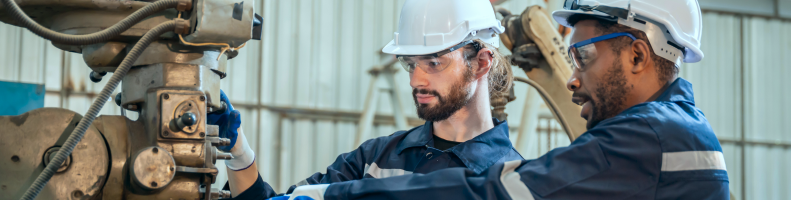
Improve customer support systems by efficiently resolving issues using AI-powered virtual assistants/chatbots.
Enhance workforce efficiency by including training programs and promote employee development programs using our advanced analytics models.
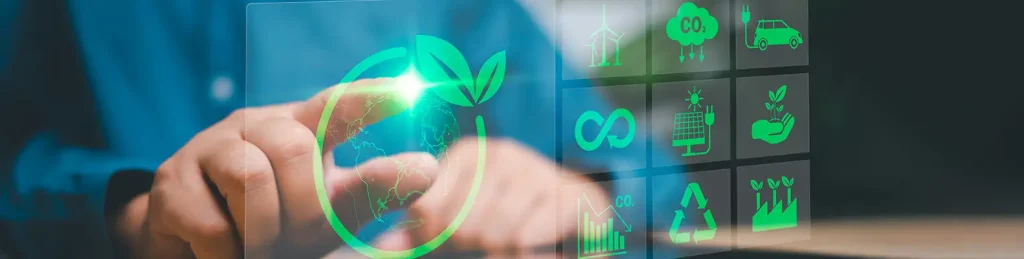
Implement dynamic pricing models based on real-time data using our data-driven solutions.
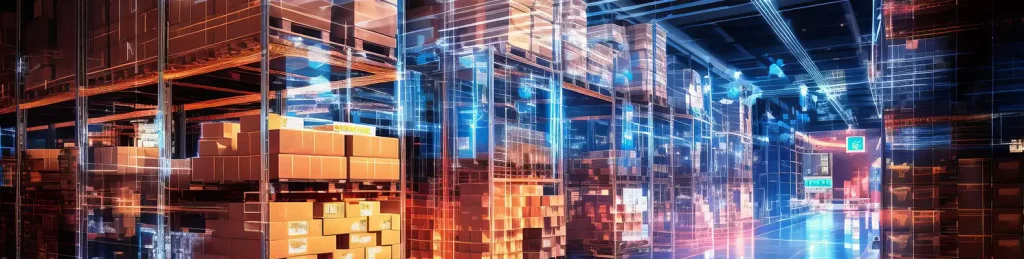
Improve overall customer experience using our predictive analytics models.
Inventory Optimization
Track order deliveries, fulfill product demands, and fix production issues for seamless manufacturing.
Sustainability
Reduce environmental footprint by implementing energy-efficient technologies and waste management practices using our sustainable solutions.
Personalized AI-proof solutions
Develop tailor-made products that meet specific customer needs using real-time market demand analysis.