What is AIOps? & Why do Businesses Fail to Take Full Advantage of AIOps Capabilities?
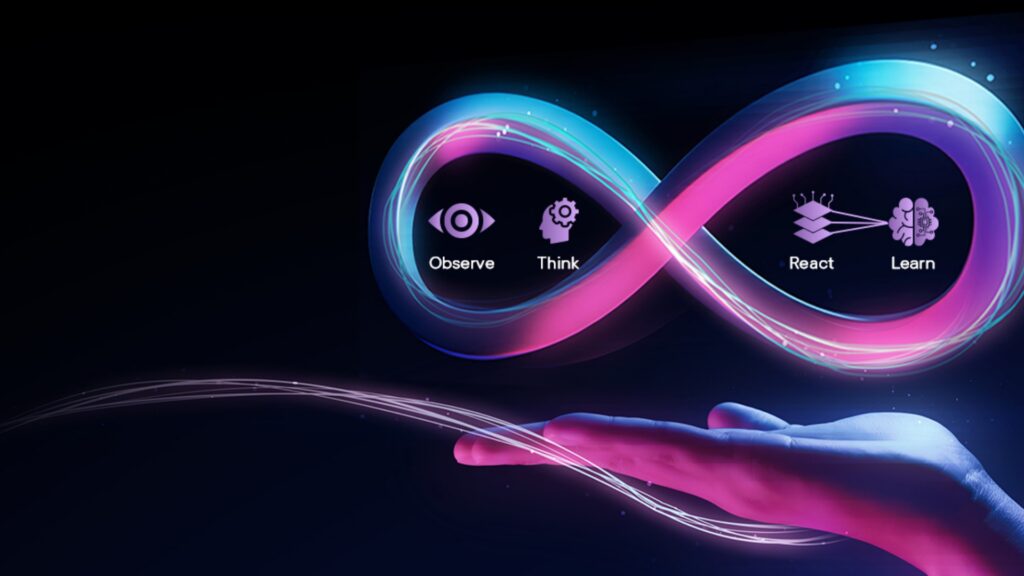
Building responsible technology has become a top priority for enterprises across industries as understanding and scrutiny of the challenges connected with AI have grown. Responsible AI evolves from a “best practice” to the “high-level principles” and guidelines required to drive system-level change, building trust around the business world. Regulators are taking notice, also advocating for regulatory frameworks surrounding AI that include enhanced data protection, governance, and reporting standards.
Can AIOps Help Your Business?
The current business environment across industries is changing rapidly, causing software and systems to grow more complicated. Cross-functional teams; are expected to generate solutions more quickly and efficiently in these circumstances. Site Reliability Engineering (SRE) and Network Operation Center (NOC) teams in the DevOps setup; are continuously flooded with a mountain of data. At the same time, these teams are increasingly under pressure to handle many dashboards, frequent alerts, and signals from multiple tools, making it harder to identify the root cause of problems/instances. AIOps as a solution; assists these teams in finding solutions to the problems more quickly and effectively, allowing them to focus more on creative work and the software development process.
Businesses are intrigued to start investing/buying an AIOps solution in order to transform their business. But factually, many business leaders and technologists fail to understand that success with AIOps lies within the right preparation and not just limited to the technology adoption.
Resilient. Agile. Ease. – “Shift Left” makes it hassle-free for SRE and NOC teams in DevOps setup
Enhancing the application development lifecycle is a potential use case for AIOps. Applications are constantly changing as a result of digital transformation, with new iterations frequently surfacing each day. A domain-agnostic solution can be “Shift Left” in the lifecycle to provide observability and control to Site Reliability Engineering (SRE) and Network Operations Center (NOC) teams in DevOps set up that were previously available to ITOM and ITSM teams.
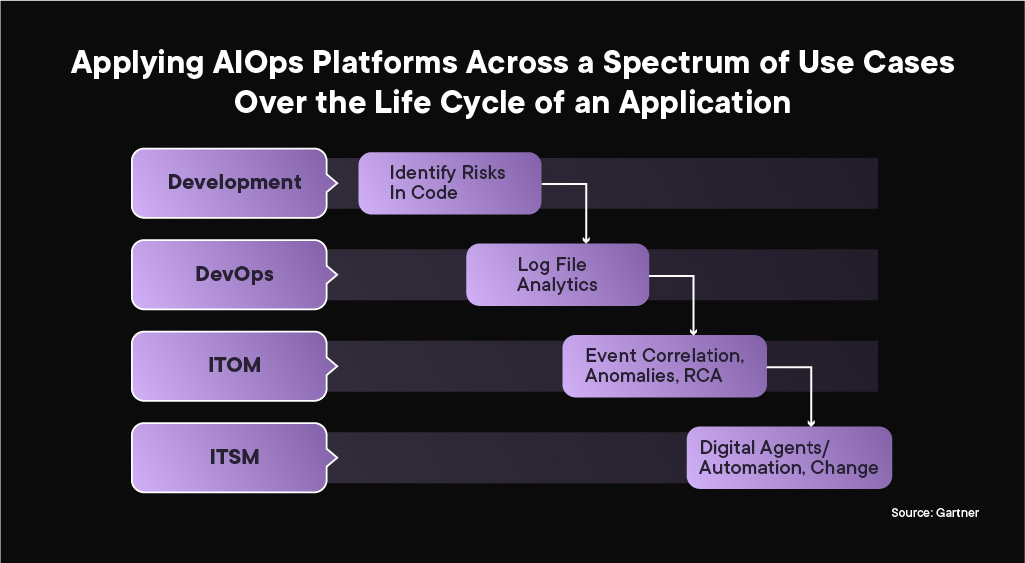
Why are businesses failing to take full advantage of AIOps capabilities?
Before discussing the bottlenecks of businesses implementing AIOps, let’s understand AIOps in a nutshell…!
What is AIOps?
– According to Gartner, “AIOps combines Big Data and Machine Learning to automate IT operations processes, including event correlation, anomaly detection, and causality determination.”
It defines artificial intelligence in IT operations and workflow. It refers to the strategic application of AI, Machine Learning (ML), and Machine Reasoning (MR) technologies across IT operations to simplify, streamline, and optimize the use of IT resources.
In a nutshell – AIOps is designed to address the growing challenges of complex IT infrastructures and help organizations make the transition to a better, more efficient future. AIOps can be used to improve IT productivity and reduce costs, automate repetitive tasks, create a more predictive environment by collecting data from multiple sources and analyzing it using ML/MR technologies, reduce time-to-market for new innovations, etc.
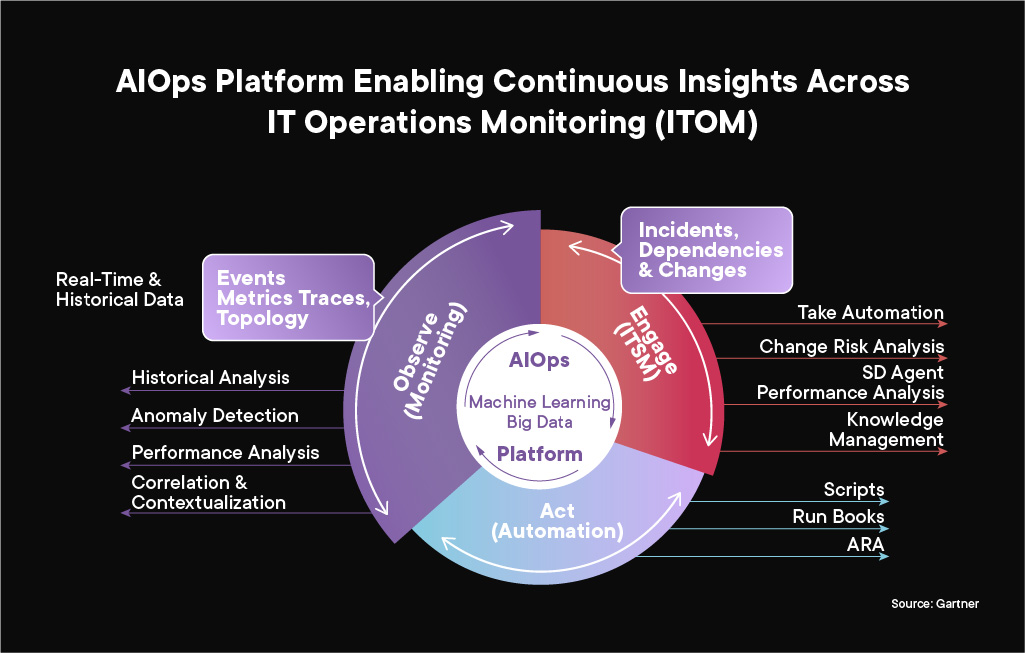
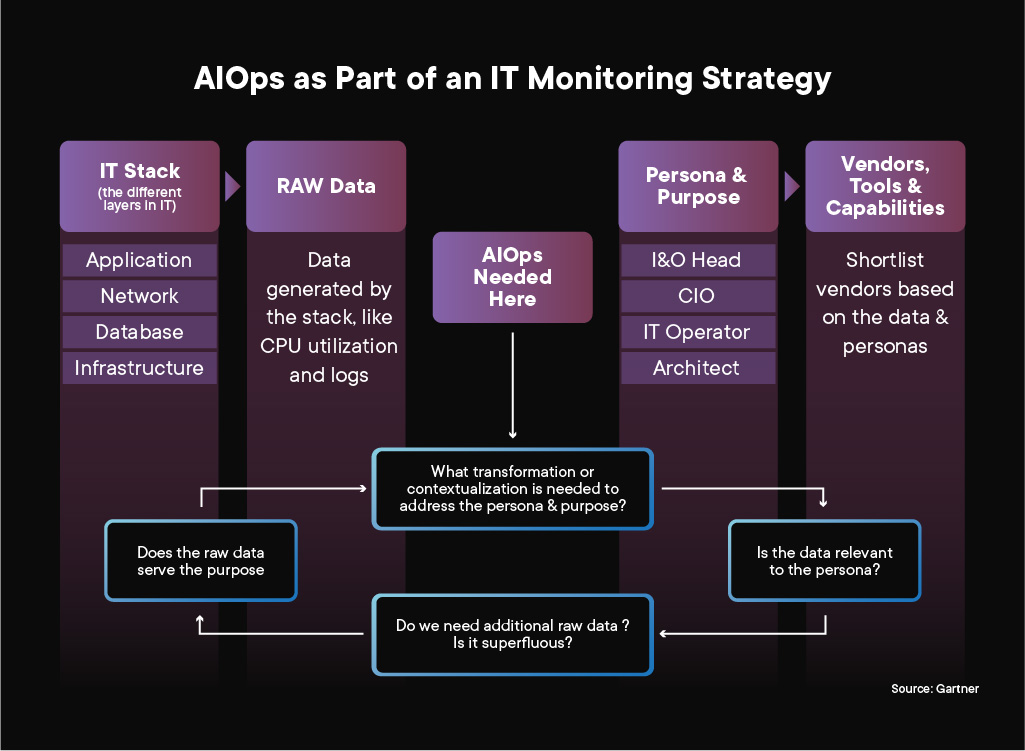
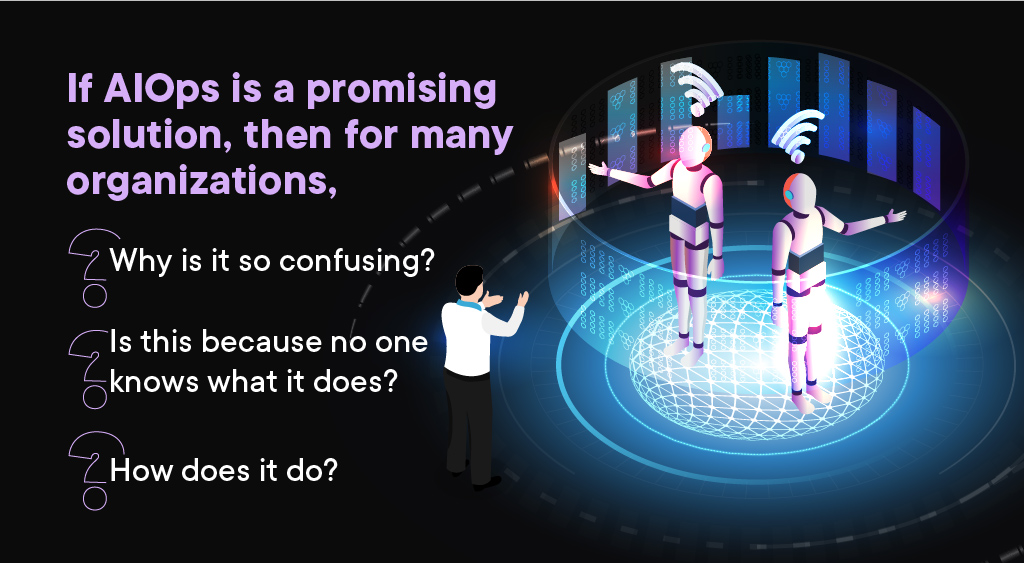
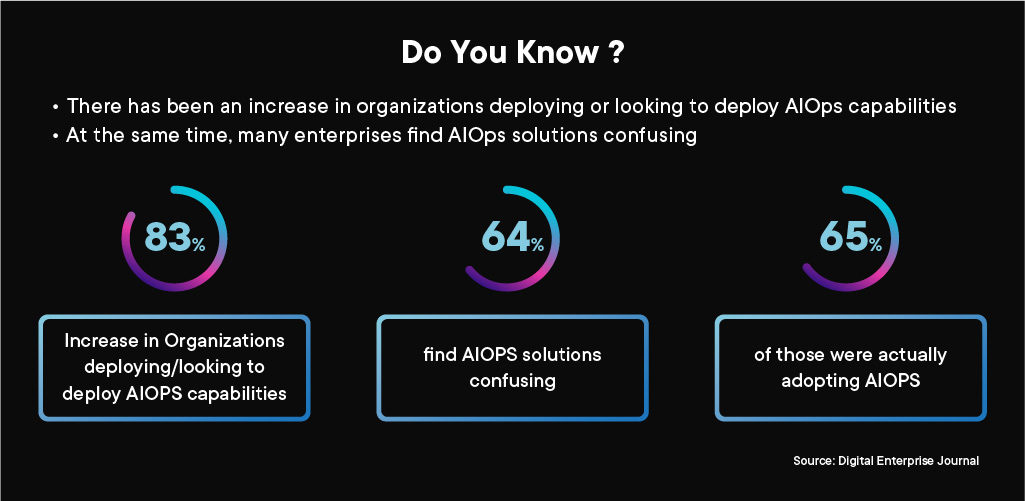
Supporting this fact are the other key findings that say –
- 53% of AI adopters mentioned “lack of transparency” as one of their significant bottlenecks
- 54% of respondents expressed concerns about making bad decisions based on AI recommendations
- 55% of respondents worried about the liability for decisions and actions taken by AI systems
And the reason they fail to implement or take full advantage of AIOps!
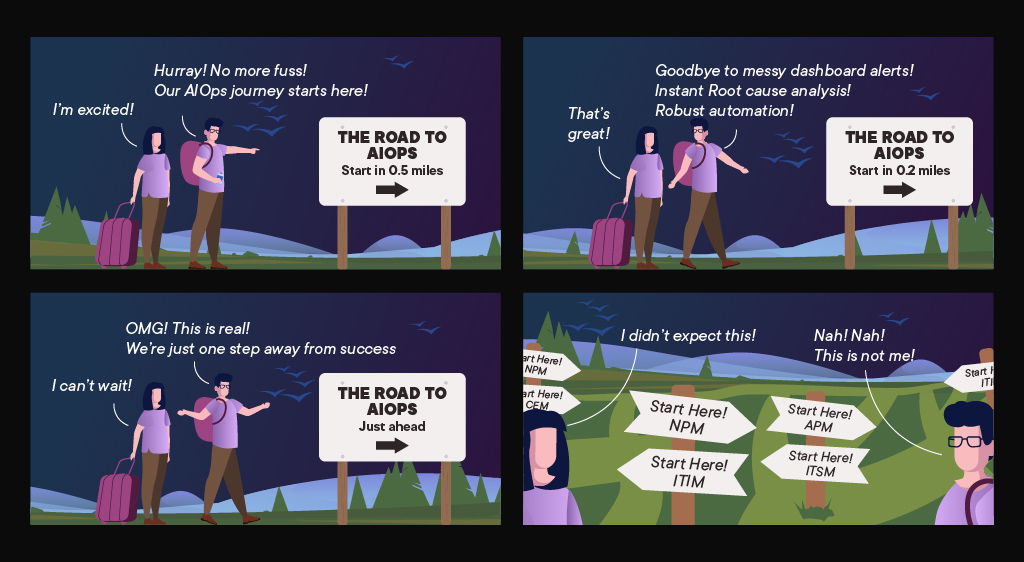
Challenges: Top 5 Reasons why AIOps implantation Could Fail
- Incompatibility with existing tools: Is it feasible for your software systems to communicate data in an efficient way? In order to provide valuable insights, an AIOps-based analytics platform will need data from other software systems. The failure of your AIOps transformation could be due to interoperability with existing software platforms.
If current software systems prevent you from collaborating with other products or systems, it’s time to think about an IT transformation. Let’s assume you are using a digital service desk AI software to generate the tickets, and your current legacy tools fail to process the request. It will land you in trouble. Thus, make sure that the tickets generated by the services desk are forwarded to your legacy tools for analysis. If your existing devices are compatible with an AIOps based analytics platform, it will automatically process the events/instances from the service desk and generate actionable insights. - Lack of awareness to identify problem areas: You aren’t undergoing AIOps transformation simply to implement cutting-edge technology. The primary objective of employing AI in operations management services is to boost the productivity of your IT operations. Apart from keeping up with the current AI trends, it would help to concentrate on the areas where an AIOps transformation is required.
Some of your IT operations may be efficient enough that you don’t need the help of an AIOps-based analytics platform. Adopting AIOps can be costly; thus, it’s best to identify the major bottlenecks that reduce the ROI (Return on Investment). Even the best AIOps tools and products have specific use cases and cannot assist you with a problem right away. - Outdated strategies to train data: In order to achieve scalable results, you need to feed the AIOps-based analytics platform training data. Data serves as fuel for AI/ML algorithms, allowing them to learn about IT processes. Organizations fail to provide training data to AIOps-based analytics platforms, resulting in AIOps transformation failure.
Even matured businesses fail to provide AI/ML algorithms with sufficient training data to improve their performance. Your AIOps-based analytics platform will not deliver relevant insights if your training data is cluttered and contains many outliers. The organization data is constantly fragmented across many software platforms and is unstructured. AIOps-based analytics tools cannot perform to their full potential without a comprehensive view of the organization’s data. - Lack of performance metrics understanding: How would you know if something is wrong with your AIOps transformation? One option is to wait and see how the attempted AIOps transformation impacts your ROI. Another method for determining the value of AIOps adoption is to use performance metrics. If you discover inefficient AI DevOps platform management services in a timely manner, you may be able to do transition to an alternative transformation plan. The following are some of the KPIs that can help in measuring the impact of AIOps transformation:
- MTTD (Mean Time to Detect): It refers to the amount of time spent investigating an IT incident. The MTTD should decrease if AI is used for application monitoring.
- MTTR (Mean Time to Detect): It signifies the amount of time it takes to resolve an IT issue. The use of AI in operations management services should always result in a considerable reduction in MTTR.
- Service availability: AIOps systems will constantly improve the availability and reliability of your services. If the availability of your services isn’t improving, it’s time to modify your AIOps strategy.
- Reluctant or inability to adapt to the changing IT culture: You need to understand that AIOps adoption is not just limited to technological changes; it will dramatically change your IT culture. For instance, it would be difficult for your cross-functional teams to trust the decisions suggested by AI data analytics monitoring tools. So, understanding its capabilities and how it works is crucial for the teams involved in the process. You need to conduct the workshops and training sessions to educate them in a timely manner. In this regard, reskilling and upskilling the respective teams could give you faster results denoting cultural shift the way teams think and work for an organizational goal. On the other hand, you can leverage open-source AI/ML tools that can be tailored to match your current IT culture.
How to overcome challenges in AIOps implementation?
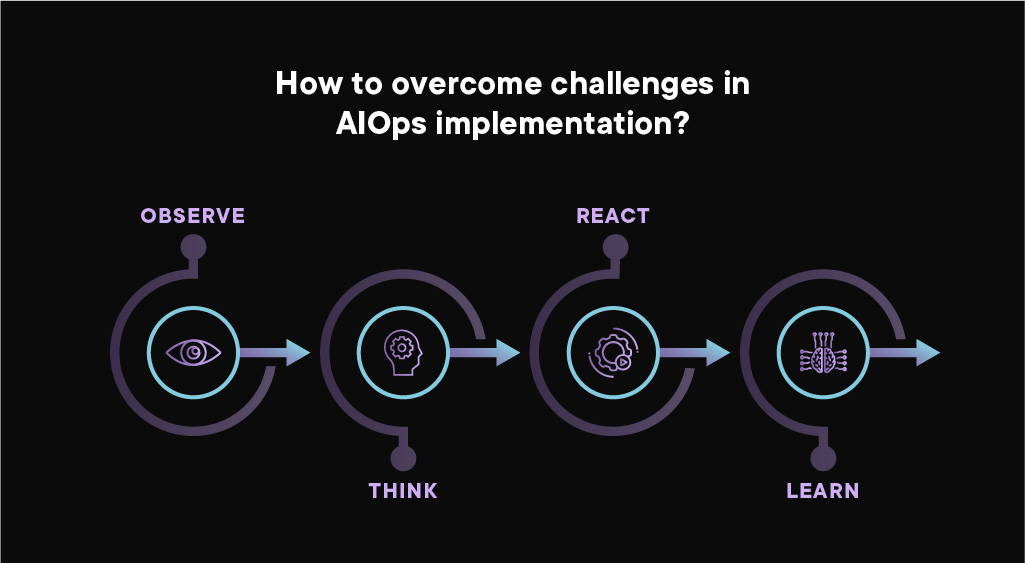
- Observe: Get started with real-time big data processing by identifying and collecting incidents/logs/alerts/request raised/event history from all underlying systems (application, network, infrastructure, etc.).
- Think: Machine learning/deep learning techniques can be used to enable AI-based insights and recommendations. Start with AI-based insights, such as noise reduction through event de-duplication and grouping and real-time anomaly detection. Event correlation for causal analysis, automated RCA, application failure prediction, and change impact analysis are some of the more advanced use cases you can consider in the process.
- React: RPA, ITPA, scripts, and orchestrators can be used to initiate auto-heal/self-remediation operations. You need to have an experienced team or train them to manage the workflows using these methodologies.
- Learn: Allow AI-based learning to learn from previous incidents from both successful/failed attempts made in the process and use it to predict future outcomes to plan your next attempt. This approach is paramount to understanding your challenges in every possible way and learning how to overcome them strategically.
AIOps Market Report & Recommendations
One of the critical factors driving market growth of AIOps is the growing demand for automation across industry verticals, such as banking, financial services, BFSI, healthcare, automation, IT, and logistics.
Businesses use AIOps to prevent and control security breaches by monitoring the activities and transactions of employees, customers, and external agencies. In accordance with this, the Covid-19 influence has resulted in widespread enterprise adoption of the work-from-home (WFH) trend, which is considerably contributing to market growth. When working remotely, AIOps is applied to improve information security and many other operational workflows effectively.
Various technological breakthroughs in cloud-computing solutions are also contributing to the AIOps growth. Businesses across industries are using cloud-based solutions for major business applications, performance, network, and security management that are gaining popularity at pace. AIOps also contributes to improving the overall efficiency of the infrastructure for service delivery with less manual efforts. In other words, considering the relentless improvements in the IT infrastructure, specifically in boosting the economies with extensive research and R&D initiatives, are expected to propel the market growth.
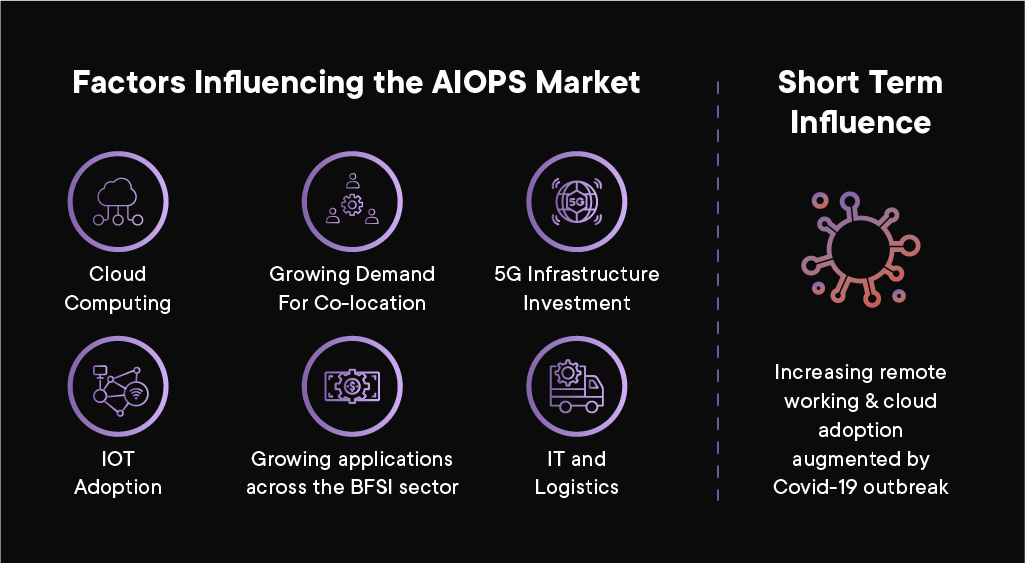
“There is no future of IT operations that does not include AIOps. This is due to the rapid growth in data volumes and pace of change (exemplified by rate of application delivery and event-driven business models) that cannot wait on humans to derive insights.”
~ Gartner
AIOps Market Size, Share and Trends – Industry Forecast 2022 to 2027
“The global AIOps market is expected to exhibit a CAGR of 21.2% during 2022-2027”
The past and current aftermath COVID-19 outbreak has given the AIOps market a significant boost. As AI-based IT operating solutions become more common, the industry has accelerated significantly, allowing businesses to reduce workflow and resource effort; allowing to focus more on innovation. During the pandemic, AIOps provided various benefits to businesses, including automating monotonous processes, delivering meaningful reporting, and improving risk management. As a result of these efforts, the industry’s post-COVID-19 repercussions have increased drastically.
AIOps Market Size, Share and Trends
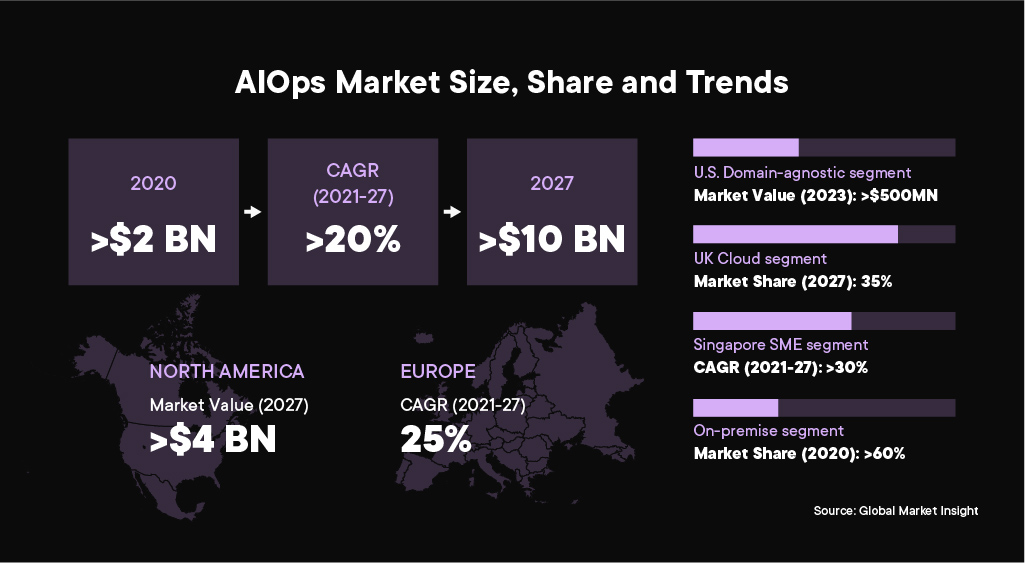
What are the AIOps trends in the U.S, Singapore & Germany? Where its market stands in these regions?
Domain-agnostic tools is the next big thing in the U.S region
In the United States, the domain-agnostic segment is expected to produce roughly USD 500 million in revenue by 2023. These solutions primarily rely on monitoring tools to collect data and respond to changing customer prerequisites. AIOps platforms are being used by businesses across the U.S to compete with and replace several traditional monitoring tools in practice. IaaS and observability monitoring is being done comprehensively within AIOps platforms, specifically if the company’s whole IT infrastructure is in the cloud.
AIOps trends and Market size in the U.S
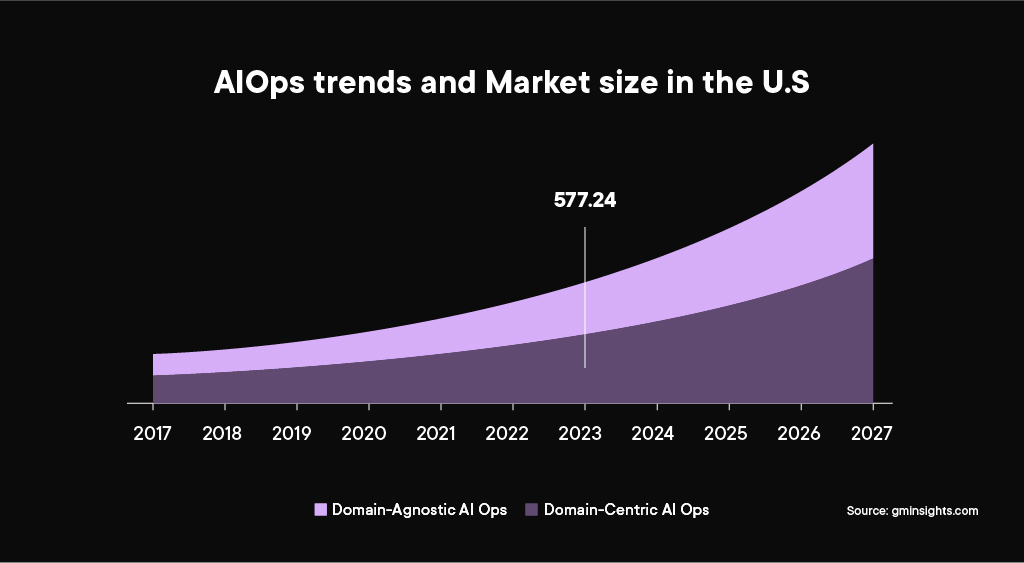
AI-based solutions to assist DevOps capabilities is gaining popularity in Singapore
The SME segment in Singapore is expected to grow at a 30% CAGR through 2027. It has been observed that SMEs are increasingly turning to AI-based IT operations tools to improve service quality and respond to changing customer needs in a more flexible manner. In contrast to this observation, below graph illustrates the enterprises vs. SMEs AIOps scenario in 2020 and its growth prediction in 2027.
Enterprises vs. SMEs – AIOps trends and Market size in Singapore
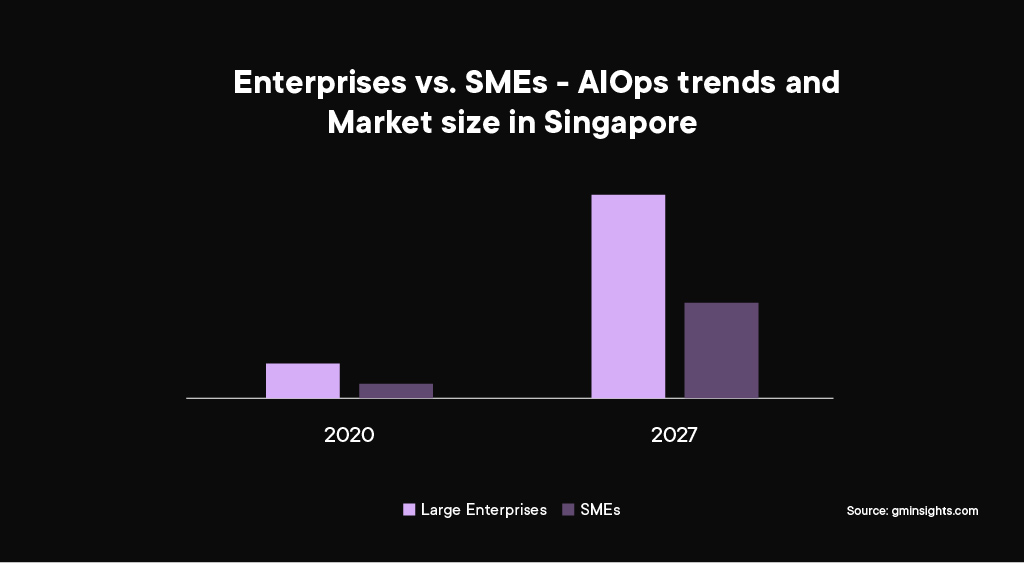
Real-time analytics and Infrastructure management are the driving force in Germany
In 2020, the real-time analytics segment in Germany amassed 35% market share followed by other segments such as application performance management, network and security management, and infrastructure management. Since then, AI is increasingly being used by businesses around the country to make insightful decisions rather than depending on human interventions, and to empower IT teams to take immediate action. The below graph denotes, by the year 2027 real-time analytics and infrastructure management sharing the equal market share.
AIOps trends, applications and market size in the U.S
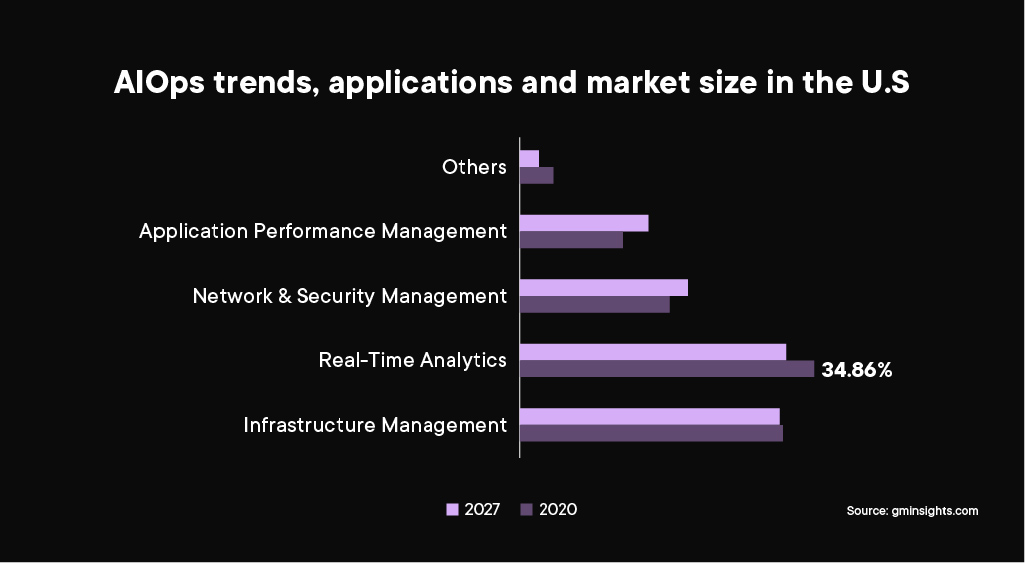
BFSI sector is expected to boost the AIOps market growth
The rapid growth and requirement of AIOps applications in the BFSI sector is one of the primary factors for the global AIOps market’s rise. As part of banking operations, employees, clients, and external agencies engage in a variety of regular and irregular activities and transactions. These operations must be closely monitored due to their complexity and confrontations. Considering these developments, AIOps is expected to boost market growth in 2022 and ahead while offering wide verity of applications to monitor real-time data and automated issue resolution.
Top 4 considerations for I&O leaders for successful AIOps implementation
I&O leaders must help their organizations become more agile and responsive to the needs of business units. The right operations services, such as monitoring and metrics, can help you continuously improve your systems’ performance and responsiveness. It should start with focusing on the development of a “change engine” within the organization, using automation to reduce manual tasks, errors and inconsistencies and leveraging technology to meet compliance requirements. Thus, these I&O leaders should focus on:
- Invest and use an incremental strategy to replace rule-based event analytics and expand into domain-centric workflows like application and network diagnostics, putting practical outcomes ahead of ambitious goals
- Allow the use case to define whether AIOps should be domain-centric or domain-agnostic. To start with use specialized use case, leverage domain-centric AIOps features built into a monitoring tool, and deploy a domain-agnostic stand-alone solution with a roadmap encompassing multiple use cases
- You need to select an AIOps platform that offers bidirectional interaction with ITSM tools to enable task automation, knowledge management, and change analysis. Ensure you’re not choosing the tools that are limited to basic search-and-display functionality
- Enable continuous insights across ITOM by following the methodology of observe, think, react and learn which is discussed in the earlier section of this article
Summing Up!
AIOps will result in a sweeping change in how AI-driven IT operations are managed. Even though the primary objective for AIOps adoption is to address operational issues arising from a highly complicated IT ecosystem and keep up with a fast-paced business environment, the ability of humans to understand and trust its insights, decisions, recommendations, and predictions will be extremely crucial.