HowStat – Application Of Data Science In Cricket
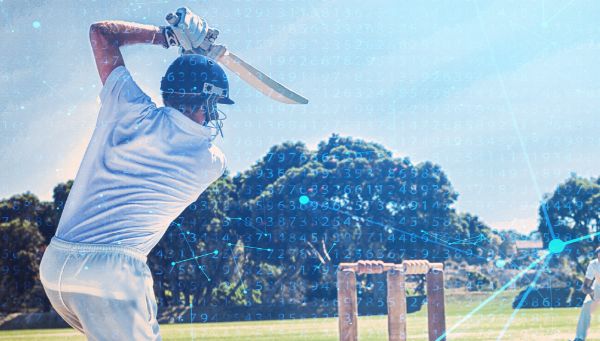
Data science helps us to extract knowledge or insights from data- either structured or unstructured- by using scientific methods like mathematical or statistical models. In the last two decades, it has been one of the most popular fields with the rise of all big data technologies. A lot of companies have been using recommendation engines to promote their products/suggestions in accordance with users’ interests such as Amazon, Netflix, Google Play. A lot of other applications like image recognition, gaming, or Airline route planning also involves the usage of big data and data science.
Sports is another field which is using data science extensively to improve strategies and predicting match outcomes. Cricket is a sport where machine learning has scope to dive into quite a large outfield. It can go a long way towards suggesting optimal strategies for a team to win a match or a franchise to bid a valuable player.
Under the International Cricket Council (ICC), there are 10 full-time member countries, 57 affiliate member countries, and 38 associate member countries, which adds up to 105 member countries. We cannot imagine the amount of data that will be generated every day for 365 days with the ball-by-ball information of 5,31,253 cricket players in close to 5,40,290 cricket matches at 11,960 cricket grounds across the world. Database maintenance has already been present in cricket for a long time back and simple analysis has also been used in the past. We have the scores of each match with all the details which have been used to generate stats like, highest run scorer, highest wicket-taker, best batting/bowling average, the highest number of centuries in away matches, best strike rate, the highest run scorer in successful chases and much more. In recent years, the depth of analysis has reached a whole new level.
The most popular use of mathematics in cricket is the Duckworth-Lewis system (D/L). The brainchild of Frank Duckworth and Tony Lewis, this method helps in resetting targets in rain-affected limited overs cricket matches. The D/L method is widely used in all limited overs international matches to predict the target score. It is a statistical formula to set a fair target for the team batting second, based on the score achieved by the first team. It takes into consideration the chasing side’s wickets lost and overs remaining. The predicted par score is calculated at each ball and is proportional to a percentage of the combination of wickets in hand and overs remaining. It is simple mathematics and has a lot of flaws. This method seems to be more beneficial for the team batting second. It does not account for changes in the proportion of the innings for which field restrictions are in place compared to a completed inning. V Jayadevan, an engineer from Kerala, also created a mathematical model alternative to the D/L method but it did not become popular because of certain limitations.
Machine Learning algorithms can be used to identify complex yet meaningful patterns in the data, which then allows us to predict or classify future instances or events. We can use data from the first innings, such as the number of deliveries bowled, wickets left, runs scored per deliveries faced and partnership for the last wicket, and compare that against total runs scored. Machine learning techniques like SVM, Neural Network, Random Forest can be used to create a model from the historical first innings data, considering the teams playing the match. The same model can be used to predict the second innings which is interrupted by rain. This will give a more accurate prediction than the D/L method, as we are using a lot of historical data and all relevant variables.
Another application is the WASP (Winning and Scoring Prediction), which has used machine learning techniques that predict the final score in the first innings and estimates the chasing team’s probability of winning in the second innings. However, this technology has been used in very few tournaments as of now. WASP was created by Scott Brooker as part of his Ph.D. research, along with his supervisor Seamus Hogan, at the University of Canterbury. New Zealand’s Sky TV first introduced the WASP during the coverage of their domestic limited overs cricket. The models are based on a database of all non-shortened ODI and 20-20 games played between top-eight countries since late 2006 (slightly further back for 20-20 games). The first-innings model estimates the additional runs likely to be scored as a function of the number of balls and wickets remaining. The second innings model estimates the probability of winning as a function of balls and wickets remaining, runs scored to date, and the target score. Let V(b,w) be the expected additional runs for the rest of the innings when b (legitimate) balls have been bowled and w wickets have been lost, and let r(b,w) and p(b,w) be, respectively, the estimated expected runs and the probability of a wicket on the next ball in that situation. The equation is –
V(b,w) =r(b,w) +p(b,w) V(b+1,w+1) +(1-p(b,w)))V(b+1,w)
Factors like the history of games at that venue and conditions on the day (pitch, weather etc.) are considered and scoring rates and probabilities of dismissals are used to make the predictions.
Other successful applications of data science in cricket are –
- “ScoreWithData”, an analytics innovation from IBM, had predicted that the South African cricketer Imran Tahir would be ranked as the power bowler, 7 hours before the first quarter final of the 2015 world cup.
South Africa went on to win the match on the back of an outstanding performance by Tahir.
- “Insights”, an interactive cricket analysis tool developed by ESPNCricInfo, is an amalgamation of cricket and big data analytics.
- In the last T20 World cup in 2016, ESPNCricInfo did some advanced statistical analysis before the start of each match, viz. when Ravichandran Ashwin takes 3 wickets, India’s chance of winning the match increases by 40%.
But, the application of data science has been used more extensively in other sports like football. The German
Football Association (DFB) and SAP had developed a “Match Insights” software system which helped the German national football team to win the 2014 World Cup. Billy Beane of “Money Ball” fame was successful by taking the drastic step of disregarding traditional scouting methods in favor of detailed analysis of statistics. This enabled him to identify the most productive players irrespective of the all-around athleticism and merchandise-shifting good looks that clubs had previously coveted.
The future of big data and machine learning is indeed very bright in the world of cricket. While the bowlers shout “Howzat” to try and clinch wickets, we as data scientists, with the help of machine learning and big data, can pose the question: HowStat?
References
- http://Reference http://www.espncricinfo.com/ci/content/page/409991.html
- http://www.computerweekly.com/news/2240224421/SAP-helps-Germany-lift-the-World-Cup
- http://theconversation.com/games-by-numbers-machine-learning-is-changing-sport-38973
- https://www.simplilearn.com/how-big-data-is-helping-teams-win-big-at-t20-world-cup-criclytics-article
- http://offsettingbehaviour.blogspot.com/2012/11/cricket-and-wasp-shameless-self.html