It’s appealing to commit all your business efforts to the forward supply chain, but the “reverse” practice is also crucial for many businesses. From coordinating defective goods returns to picking up empty packaging to collecting old household appliances and other used products, these operations necessitate a streamlined and effective reverse supply chain.
Why would a business need to implement and then reverse a supply chain? Isn’t it perplexing? Let’s dive right into the details.
What is a reverse supply chain?
It’s the series of activities to retrieve a used product/part from a customer, either dispose of it or reuse it. And for a growing number of manufacturers in industries ranging from handbags to supercomputers, the reverse supply chain has become an essential part of their business.
In some regions of the world, companies are forced to set up reverse supply chains because of ESG or consumer pressures.
Let’s consider Kodak; it remanufactures its single-use cameras after the film has been developed. Over the past 10 years, the company has recycled millions of cameras in more than 20+ countries. Few companies are using reverse supply chains as an integral part of new and existing businesses, such as Bosch.
When a company establishes a reverse supply chain by choice or necessity, it faces many challenges. It should educate its customers and establish new points of contact with them, decide what activities to outsource and what to do, and figure out how to keep costs to a minimum while discovering innovative ways to recover value.
Key Components of the Chain
To understand the structure of a reverse supply chain, let’s divide the chain into its five key components:
Acquisition:
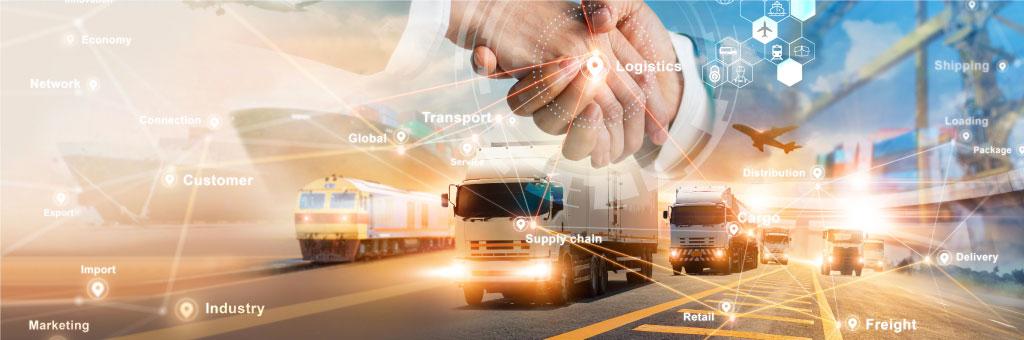
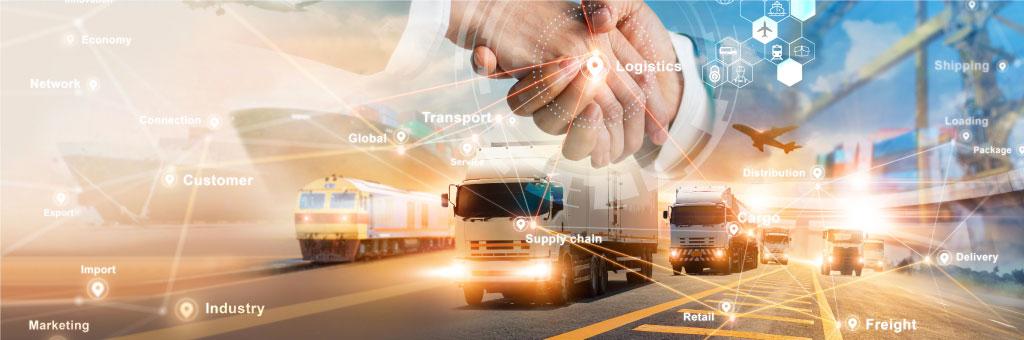
The task of retrieving used products is key to creating a profitable chain. Product returns’ quality, quantity, and timing need to be carefully understood and managed. Furthermore, companies may be flooded with returned products of such variable quality that they make remanufacturing impossible. Companies often work closely with retailers and distributors to coordinate collections.
Reverse Logistics:
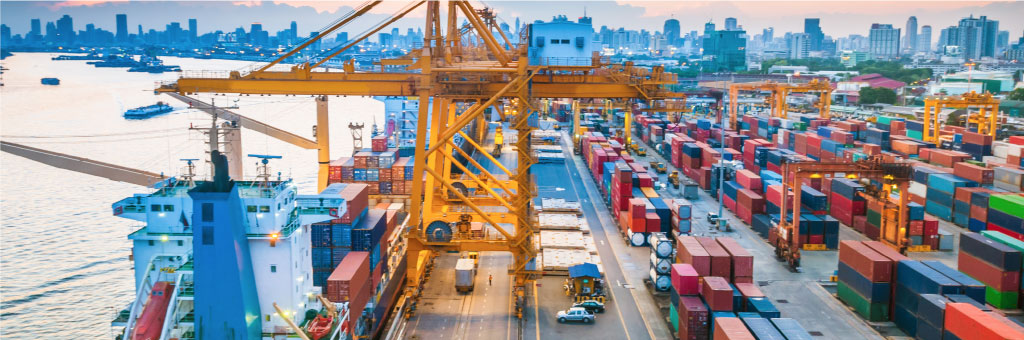
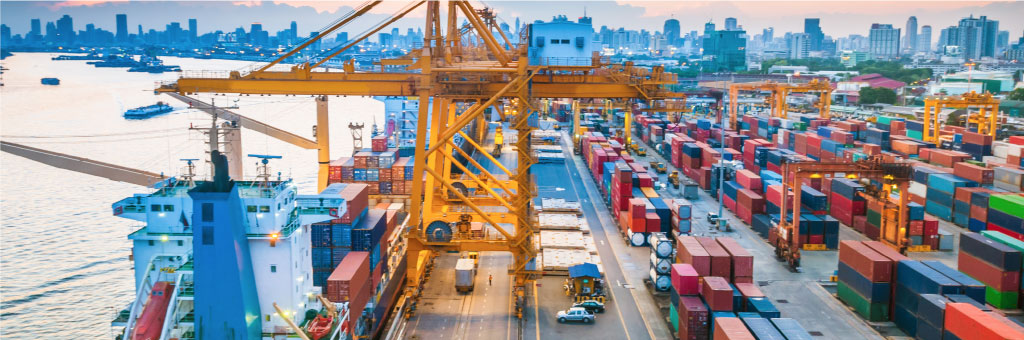
Once collected, the products need to be transported to facilities for various processes such as inspection, sorting, and disposition. There is no one “best” design for a reverse logistics network; each must be tailored to the products involved and the economics of their reuse. Bulky products like refrigerators, for instance, will require very different handling than small but fragile products like cameras, lenses, etc. Companies consider not only the costs for shipping and storage but also how quickly the value of the returned products will decline and the need for control over the products. In many cases, companies outsource the logistics to a specialist, which makes better sense in some scenarios.
Sort, Sweep & Segregate(3S):


The check, sort, sweep, segregate, and grading of returned products are labor-intensive and time-consuming tasks. But the process can be streamlined if a company subjects the returns to quality standards and uses technologies to automate (such as sensors, bar codes, etc.) to track and test them. A business should learn to make 3S decisions based on quality, product spec, or other variables at the earliest possible stage during the return process. This helps eliminate many logistics costs and gets remanufactured products to market faster.
Refurbishment – Renovation:
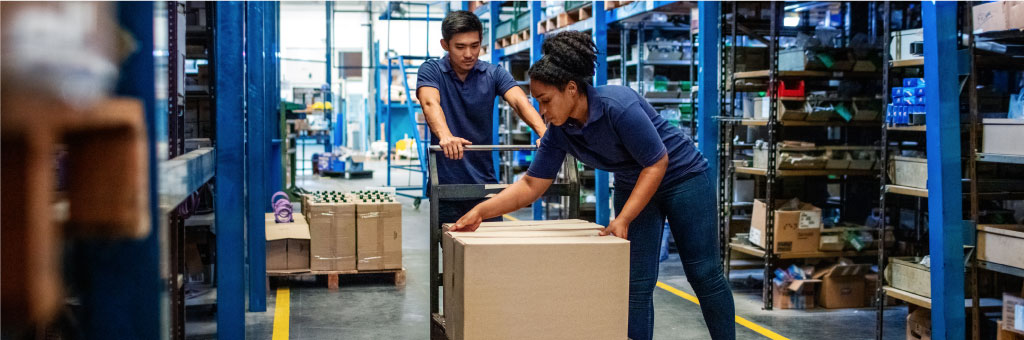
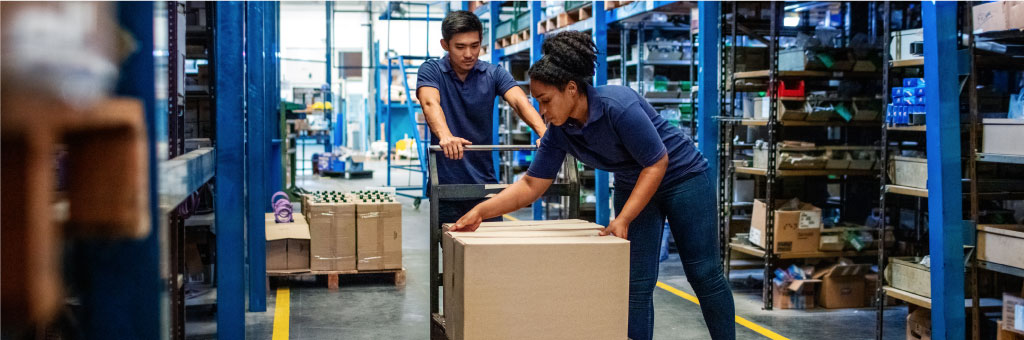
Companies may capture value from returned products by extracting and reconditioning components for reuse or remanufacturing the products for resale. Reconditioning and reproduction processes are much less predictable than traditional manufacturing because there can be a large degree of uncertainty in the timing and quality of returned products. Making smart decisions while accepting and sorting returns will help largely reduce manufacturing variability and costs.
Market Re-entry:
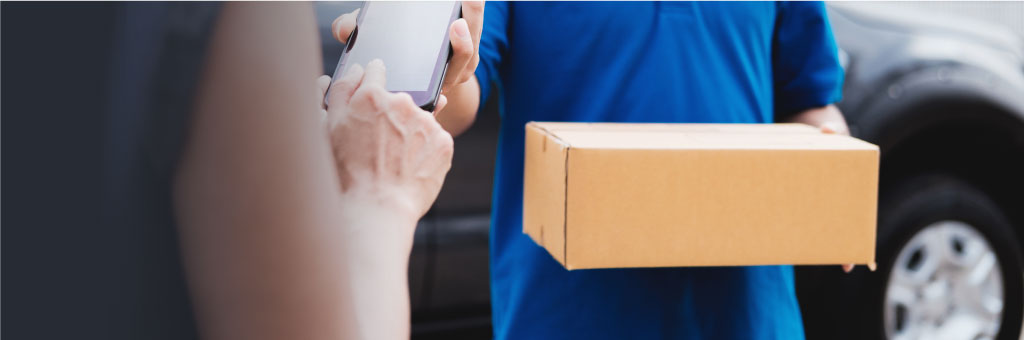
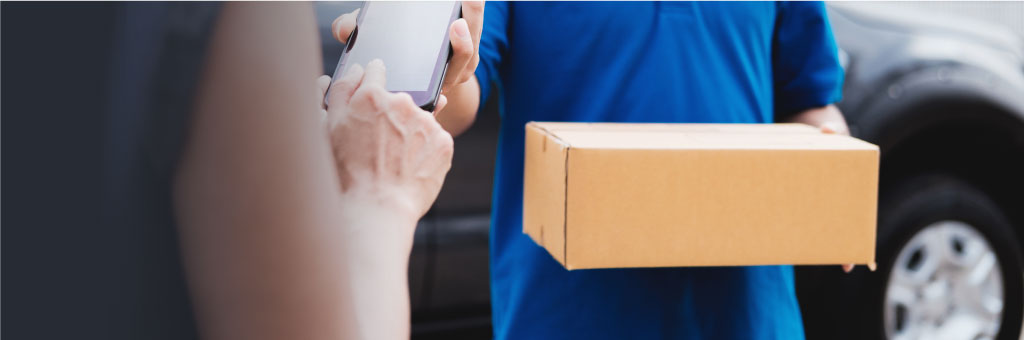
If any company plans to sell a recycled product, it first determines whether there is a demand for it or if a new market must be created. The company needs to invest heavily in consumer education and other marketing efforts if it’s the latter. Potential customers for remanufactured products or components include not just the original purchasers but also new customers in different markets. The company will sometimes target customers who cannot afford the new products but would jump at the chance to buy used versions at lower prices.
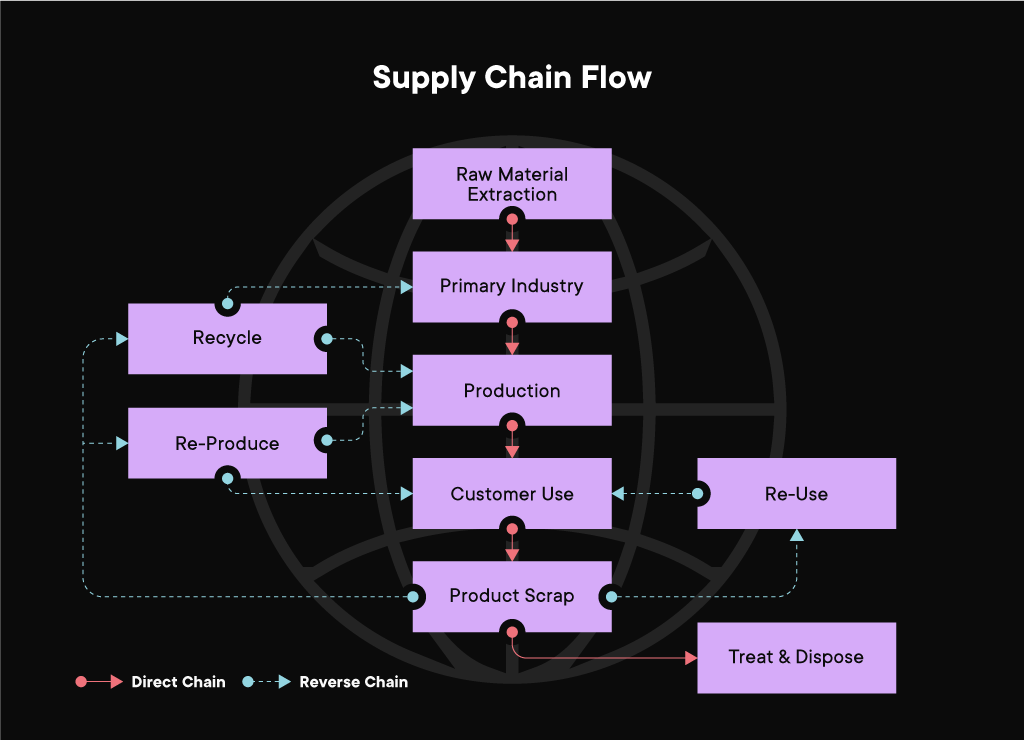
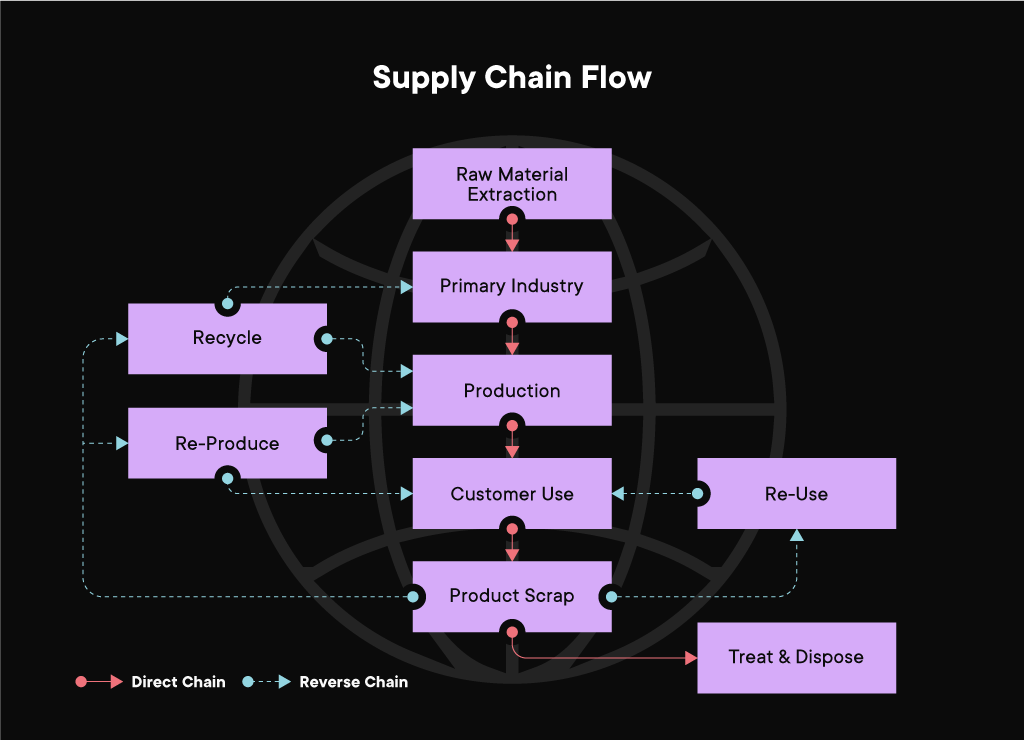
Artificial Intelligence for Reverse Supply Chain
Using AI for reverse supply chain management enables businesses to address waste management and environmental sustainability issues.
The reverse supply chain is a part of a more significant concept known as the circular economy, which seeks to tackle problems such as waste reduction, pollution, biodiversity loss, and climate change. Considering sustainability, governing bodies around the world create product take-back laws, which hold manufacturers financially accountable for factors related to waste recycling, handling of perishable goods, and to produce circular products. Managing these activities effectively offers businesses a more significant challenge in reverse logistics. Using new approaches and tools like AI for reverse supply chains can help overcome the challenges associated with reverse logistics.
To optimize the performance of the reverse supply chain, there is a need to establish an effective and efficient infrastructure via optimal network design. Generally, reverse supply chain network design is concerned with establishing an infrastructure to manage the reverse channel, which often consists of final users, collectors, and remanufacturers.
Using Artificial Intelligence (AI) for reverse logistics offers a great range of solutions to get the world closer to the ideal circular economy. Artificial Intelligence driven data analytics, image processing, and other techniques can simplify reverse logistics.
Classifying Waste Materials
Artificial Intelligence based waste recognition systems feature image classification and processing to remove inconsistencies from the process of material sorting and composition analysis. Thus, waste materials’ identification and categorization are optimized to maximize recycled goods’ quality. Artificial Intelligence-based machine vision recycling systems enables waste managers and recycling department to receive valuable insights to increase the recycling rates and product value. Machine vision-aided image processing holds the potential to add new dimensions to the waste management and product recycling processes. Using 2D and 3D imaging, Artificial Intelligence allows the businesses to optimize waste material segregation.
Repurposing Returned Goods
Automated analytics and AI are potent tools for repurposing goods that your customers have returned. So, the business can choose whether it must liquidate, re-shelve, recycle, or scrap a returned product after an Artificial Intelligence-based tool assesses and predicts the usability and condition of such goods. Additionally, Artificial Intelligence also predicts and provides recommendations about how businesses can dispose of returned products at the point of return. So, selecting the best option for processing a returned item can be automated, thereby increasing its speed and effectiveness in decision-making and reducing the expenses incurred for each returned item.
Artificial Intelligence can also be deployed to reroute products in reverse logistics that their buyers have returned. Using its decision-making tools and inventory management systems, assess a returned product’s condition and various other factors, such as demand for the same product in other regions, before recommending to the sales team to market the product in such areas.
Designing Circular Products
Artificial Intelligence helps speed up circular products’ overall design and development processes to make them arrive in commercial markets sooner. Machine learning-based systems produce components, materials, and products that fit the ideal circular economy. Artificial Intelligence enables designers to select product designs faster as the technology analyzes large amounts of data and quickly suggests optimal designs and their adjustments. Once the base design with various tweaks and changes is suggested, the product designer can start creating the product that will be the most sustainable and recyclable. The insights generated by Artificial Intelligence are responsible for manufacturers creating circular products quickly in the most cost-effective way.
Reverse Supply Chain Use Cases
- The best example of a business leveraging Artificial Intelligence to resell its returned goods is the return management strategy by IKEA. The renowned conglomerate deployed an Artificial Intelligence-based solution to ensure that the large and expensive merchandise returned is not wasted. It has been found that one in every ten products comes back from being sold by the Swedish company globally. To reduce the losses, IKEA chose Oporto, a specialized reverse logistics-based software company, to design a predictive Artificial Intelligence tool that provides suggestions backed by data for the best possible destination for returned merchandise. The Artificial Intelligence tool allows IKEA’s product managers to know whether a returned product should be sold to a third-party retailer, donated to charity, or brought back to the floor.
- H&M is a clothing brand that has used the concept of “Reverse Logistics” innovatively. H&M accepts old clothes of any brand. They take the used clothes to create their all-recycled clothing line. The idea behind this is to connect people with the brand by selling to them and making them involved with the brand by giving away their old clothes.
- Apple manufactures various products, such as the iPhone, MacBook, etc., and sells them in its stores worldwide. Apple lets its customers return their old Apple phones when they want to upgrade to newer ones. They take their customers’ old phones and provide a discount on the new ones. Apple takes the old phones back to their factories and uses the parts of the old phones to manufacture new phones. This not only makes them more profit but also helps manufacture products in an environmentally friendly way.
- Amazon is one of the largest e-commerce websites and a pioneer in selling online. Initially, it was difficult for e-commerce websites to gain customers’ trust. Hence, Amazon started a free-of-cost product return and replacement policy on certain products only under specific conditions & also when the customers have a genuine reason to return products. Amazon handles its reverse logistics process through various third-party vendors and organic resources such as Genco, FedEx, and many other small vendors. This way, they gain their customers’ trust and ensure that their sellers provide good quality products to the customers so that fewer returns occur.
Few KPI’s considered
- Disposition Cycle Time: Cycle time is the total time it takes to move a unit from the beginning to the end of a physical process.
- Handling Cost: Handling costs are the cost of holding products in the inventory.
- Cost of Repair or Refurbishment: Understanding the cost of repair or refurbishment is a common KPI that is measured.
- Rate of Recovery: How much effort did you spend repairing the item? How long did it take for the item to return to stock? How much did it sell for on an online eCommerce channel? The recovery rate will show you how much value you recovered after selling the item.
Final Words
Companies that have had the most success with their reverse supply chains are those that precisely synchronize them with their forward supply chains, resulting in what is known as a closed-loop system. The approach significantly reduces inspection and disposal expenses, allowing the business to profit from remanufactured tools. Forward-thinking yields significant returns, even in reverse supply chains.
Schedule a call today to learn more about our success stories and capabilities in the Manufacturing sector.